Növekedés/Személyre szabott első nap/Strukturált feladatok/Hivatkozás hozzáadása
Add a link
![]() Suggest potential wikilinks as easy edits for newcomers
|
Ez az oldal a Növekedési csapat munkáját írja le a "hivatkozás hozzáadása" strukturált feladattal kapcsolatban, ami a strukturált feladat egyik típusa, melyet a Növekedési csapat a Kezdők kezdőlapja segítségével kínál. Ez az oldal tartalmazza a főbb eszközöket, terveket, nyitott kérdéseket és döntéseket. Az előrehaladásról szóló legtöbb apró frissítés az általános Növekedési csapat frissítések oldalára kerül, néhány nagyobb vagy részletes frissítés pedig ide.
2021 augusztusától a feladat első iterációját az arab, cseh, vietnámi, bengáli, lengyel, francia, orosz, román, magyar és perzsa Wikipédiában létrehozott új felhasználói fiókok feléhez telepítik. A funkció bevezetésének első két hetének adatait elemeztük, és azt találtuk, hogy a kezdők sok ilyen szerkesztést végeznek, és hogy alacsony a visszaállítási arányuk. Az elemzés tanulságai alapján javítottuk a funkciót, és az eredmények arra ösztönöznek bennünket, hogy a funkciót több wikire is kiterjesszük.
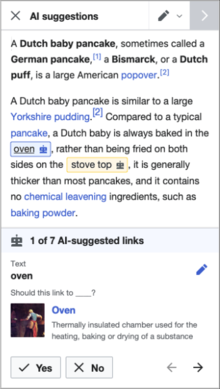
Ezekben az interaktív prototípusokban láthatod, hogy min dolgozunk. Megjegyzendő, hogy mivel ezek prototípusok, nem minden gomb működik:
A csapat tagjai a 2021-es Wikimánián bemutatták a munka hátterét, algoritmusát, megvalósítását és eredményeit. Nézd meg a videót itt. and the diafilmek itt.
Jelenlegi állapot
- 2020-01-07: a hivatkozás ajánló algoritmus kivitelezhetőségének első értékelése
- 2020-02-24: a továbbfejlesztett hivatkozás ajánló algoritmus értékelése
- 2020-05-11: közösségi vita a strukturált feladatokról és az ajánlások összekapcsolásáról
- 2020-05-29: kezdeti wireframe-ek
- 2020-08-27: backend tervezés kezdetét veszi
- 2020-09-07: a mobiltervek felhasználói tesztelésének első fordulója
- 2020-09-08: felhívás a legújabb tervek közösségi megvitatására
- 2020-10-19: a mobiltervek felhasználói tesztelésének második fordulója
- 2020-10-21: az asztali tervek felhasználói tesztelésének első fordulója
- 2020-10-29: a frontend tervezés kezdetét veszi
- 2020-11-02: a szerkesztői tesztelés második fordulója az asztali tervek tesztelésében
- 2020-11-10: felhívás az arab, vietnámi és cseh közösségek visszajelzésére a tervekkel kapcsolatban
- 2021-04-19: a terminológiáról és a mérésről szóló szakaszok hozzáadása
- 2021-05-10: a funkció a négy kísérleti wikin már tesztelés alatt áll a termelésben
- 2021-05-27: az arab, vietnámi, cseh és bengáli Wikipédiákon a kezdők felének bevetése
- 2021-07-21: a lengyel, az orosz, a francia, a román, a magyar és a perzsa Wikipédián a kezdők felét alkalmazzák.
- 2021-07-23: a funkció bevezetésének első két hetéről készült elemzés.
- 2021-08-15: prezentáció a Wikimánián a háttérről, a megvalósításról, az algoritmusról és az eredményekről.
- 2022-05-20: A funkció 2. Iterációjának befejezése, mely a közösségi visszajelzéseken és az adatelemzésen alapuló fejlesztéseket tartalmaz (lásd a fejlesztések listáját a Hivatkozás hozzáadása "Iteration 2" oldalon.
- 2022-06-19: Közzététel "hivatkozás hozzáadása" kísérleti elemzés
- 2022-08-20: munka megkezdése a strukturált feladatokkal kapcsolatos járőrök visszajelzéseinek kezelésére (T315732)
- 2022-09-02: az új szerkesztők feladatainak szerkesztési típuselemzésének közzététele
- 2023-12-31: Almost all Wikipedias now have this task available for newcomers. The exception are a dozen of small wikis where there are not enough articles to activate the algorithm, and English and German Wikipedias.
- Következő: az összes wikire való telepítés és a járőrökkel való együttműködés, hogy javuljon a hivatkozás szerkesztésével kapcsolatos tapasztalat.
Összefoglaló
A Struktúrált feladatok célja, hogy a szerkesztési feladatokat lépésről lépésre olyan munkafolyamatokra bontja, melyek értelmesek a kezdők számára és mobil eszközökön is. A növekedési csapat úgy véli, hogy az újfajta szerkesztési munkafolyamatok bevezetése lehetővé teszi, hogy több új szerkesztő vegyen részt a Wikipédia szerkesztésében, akik közül néhányan megtanulnak majd jelentősebb szerkesztéseket végezni és bekapcsolódni a közösségi életbe. Miután megbeszéltük a strukturált feladatok ötletét a közösségekkel, elhatároztuk, hogy létrehozzuk az első strukturált feladatot: "hivatkozás hozzáadása". Ez a feladat egy algoritmus segítségével mutat rá olyan szavakra vagy kifejezésekre, melyek jó wikilinkek lehetnek, és a kezdők elfogadhatják vagy elutasíthatják a javaslatokat. Ezzel a projekttel szeretnénk tapasztalatokat gyűjteni ezekről a kérdésekről:
- A kezdők számára érdekesek a strukturált feladatok?
- Vajon vannak-e sikereik a kezdőknek a strukturált feladatokkal mobilon?
- Generálnak-e értékes szerkesztéseket?
- Arra késztetnek-e egyes kezdőket, hogy növeljék részvételüket?
A "Hivatkozás hozzáadása" kísérlet elemzése befejezése után megállapíthatjuk, hogy a strukturált "hivatkozás hozzáadása" feladat javítja a kezdők eredményeit mind a kontrollcsoporthoz képest, amely nem férhetett hozzá a Növekedési funkciókhoz, mind pedig a strukturálatlan "hivatkozás hozzáadása" feladatot kapó csoporthoz képest, különösen a "konstruktív" (nem visszafordított) szerkesztések esetében. A legfontosabb pontok a következők:
- Azok a kezdők, akik megkapják a Hivatkozás hozzáadása strukturált feladatot, nagyobb valószínűséggel aktiválódnak (azaz elvégzik az első cikkszerkesztést).
- Emellett nagyobb valószínűséggel maradnak meg (azaz visszatérnek, és egy másik napon egy másik szócikket szerkesztenek).
- A funkció emellett növeli a szerkesztési mennyiséget (azaz az első néhány hétben végzett szerkesztések számát), ugyanakkor javítja a szerkesztés minőségét (azaz annak valószínűségét, hogy a kezdők szerkesztéseit visszaállítják).
Miért a wikilinkek?
Az alábbi részlet a strukturált feladatok oldalról származik, mely elmagyarázza, hogy miért választottuk a "hivatkozás hozzáadása" feladatot az első strukturált feladatként.
A növekedési csapat jelenleg (2020 májusában) a "hivatkozás hozzáadása" munkafolyamatot szeretné előnyben részesíteni a fenti táblázatban felsorolt többi munkafolyamathoz képest. Bár más munkafolyamatok, mint például a "copyedit", értékesebbnek tűnnek, számos okunk van arra, hogy először a "hivatkozás hozzáadásával" kezdjük:
- Rövid távon a legfontosabb dolog, amit először is szeretnénk tenni, hogy bebizonyítsuk, hogy a "strukturált feladatok" működhetnek. Ezért a legegyszerűbbet szeretnénk megépíteni, hogy a szerkesztőkhöz telepíthessük és ismereteket gyűjthessünk, anélkül, hogy túl sokat kellene befektetnünk az első verzióba. Ha az első verzió jól megy, akkor bátran fektethetünk a nehezebben építhető feladattípusokba.
- A "hivatkozás hozzáadása" tűnik a legegyszerűbbnek, mert már létezik egy algoritmus, melyet a WMF kutatócsoport épített, és ami úgy tűnik, hogy jó munkát végez a wikilinkek felajánlásában (lásd az Algoritmus részt).
- A wikilink hozzáadása általában nem igényli, hogy a kezdők bármit is beírjanak, ami szerintünk különösen egyszerűvé teszi számunkra a tervezést és az elkészítést -- valamint a kezdők számára is.
- A wikilink hozzáadása alacsony kockázatú szerkesztésnek tűnik. Más szóval, egy szócikk tartalma nem sérülhet annyira a hivatkozások helytelen hozzáadásával, mint a források vagy képek helytelen hozzáadásával.
Tervezés
Ez a rész tartalmazza a jelenlegi tervezési gondolkodásunkat. A "hivatkozás hozzáadása" strukturált feladat tervei körüli teljes gondolatmenetet lásd ezen a diavetítésen, mely a hátteret, a szerkesztői történeteket és a kezdeti tervezési koncepciókat tartalmazza.
A terveink több körös szerkesztői tesztelésen és iteráción keresztül alakultak ki. 2020 decemberében döntöttünk azokról a tervekről, melyeket a funkció első verziójához tervezünk. Ezekben az interaktív prototípusokban láthatod azokat. Megjegyzendő, hogy mivel ezek prototípusok, nem minden gomb működik:
Összehasonlító felülvizsgálat
Amikor egy funkciót tervezünk, megnézzük a Wikimédia világán kívüli más szoftverplatformok hasonló funkcióit. Az alábbiakban az Android javasolt szerkesztési funkciójának előkészítésekor végzett összehasonlító felülvizsgálatokból mutatunk be néhány kiemelést, melyek továbbra is relevánsak a projektünk szempontjából.
- Task types – are divided into five main types: Creating, Rating, Translating, Verifying content created by others (human or machine), and Fixing content created by others.
- Visual design & layout – incentivizing features (stats, leaderboards, etc) and onboarding is often very visually rich, compared to pared back, simple forms to complete short edits. Gratifying animations often compensate for lack of actual reward.
- Incentives – Most products offered intangible incentives grouped into: Awards and ranking (badges) for achieving set milestones, Personal pride and gratification (stats), or Unlocking features (access rights)
- Users motivations – those with more altruistic motivations (e.g., help others learn) are more likely to be incentivized by intangible incentives than those with self-interested motivations (e.g., career/financial benefits)
- Personalization/Customization – was used in some way on most apps reviewed. The most common customization was via surveys during account creation or before a task; and geolocalization used for system-based personalization.
- Guidance – Almost all products reviewed had at least basic guidance prior to task completion, most commonly introductory ‘tours’. In-context help was also provided in the form of instructional copy, tooltips, step-by-step flows, as well as offering feedback mechanisms (ask questions, submit feedback)
Initial wireframes
After organizing our thoughts and doing background research, the first visuals in the design process are "wireframes". These are simply meant to experiment and display some of the ideas we think could work well in a structured task workflow. For full context around these wireframes, see the design brief slideshow.
-
Giving more explanation the first time the newcomer uses the workflow than on subsequent times
-
Explaining why the task is worth doing
-
Teaching concepts through checklists
-
Letting users disagree with algorithms and generate useful data in the process
-
Encouraging the newcomer to move on to more impactful tasks and learn more
-
Providing opportunities to learn the more powerful editing tools
-
Guardrails against low-quality contributions
-
Rewarding good work
Mobil modellek: 2020. augusztus
Csapatunk megvitatta az előző szakaszban szereplő vázlatokat. Mérlegeltük, hogy mi lenne a legjobb a kezdőknek, figyelembe véve a közösség tagjai által kifejezett preferenciákat, és gondolkodtunk a tervezési korlátokról. 2020 augusztusában megtettük a következő lépést, és maketteket készítettünk, melyek célja az volt, hogy részletesebben megmutassuk, hogyan nézhet ki a funkció. Ezeket a maketteket (vagy hasonló változatokat) a csapat megbeszélései, a közösségi megbeszélések és a szerkesztői tesztek során fogjuk használni. Az egyik legfontosabb dolog, amire ezekkel a modellekkel kapcsolatban gondoltunk, az az aggodalmunk, amit a közösség tagjaitól folyamatosan hallottunk a vita során: a strukturált feladatok alkalmasak lehetnek arra, hogy a kezdők megismerkedjenek a szerkesztéssel, de azt is szeretnénk, hogy a hagyományos szerkesztőfelületeket is megtalálják és használni tudják, amennyiben érdekli őket.
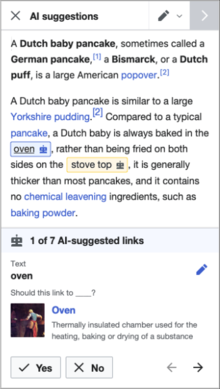
Két különböző tervezési koncepcióhoz van makettünk. Nem feltétlenül az a célunk, hogy egyik vagy másik tervezési koncepciót válasszuk. A két koncepció inkább a különböző megközelítések bemutatására szolgál. A végleges terveink tartalmazhatják mindkét koncepció legjobb elemeit:
- A koncepció: a strukturált feladatszerkesztés a vizuális szerkesztőben történik. A szerkesztő láthatja a teljes szócikket, és az "ajánló módból" forrás- vagy vizuális szerkesztő módba válthat. Kevésbé fókuszál a hivatkozások hozzáadására, de könnyebb hozzáférést biztosít a vizuális és a forrásszerkesztőhöz.
- B koncepció: a strukturált feladat szerkesztése egy saját új területen történik. A szerkesztőnek csak a szócikknek azt a bekezdését mutatja meg, mely a figyelmét igényli, és ha úgy dönt, szerkesztheti a szócikket. Kevesebb zavaró tényező a hivatkozások hozzáadása, de távolabbi hozzáférés a vizuális és forrásszerkesztőkhöz.
Felhívjuk a figyelmet arra, hogy ebben a modellsorozatban a hangsúly a szerkesztői folyamaton és az élményen van, nem pedig a szavakon és a nyelvezeten. Csapatunk végigmegy egy folyamaton, hogy meghatározzuk, hogyan lehet a legjobban leírni a szavakat a funkcióban, és elmagyarázzuk a szerkesztőnek, hogy kell-e hivatkozást hozzáadni.

Static mockups
Ha szeretnéd megtekinteni ezeket a tervezési koncepciókat, javasoljuk, hogy tekintsd meg az alábbi teljes diakészletet.

Interactive prototypes
You can also try out the "interactive prototypes" that we're using for live user tests. These prototypes, for Concept A and for Concept B, show what it might feel like to use "add a link" on mobile. They work on desktop browsers and Android devices, but not iPhones. Note that not everything is clickable -- only the parts of the design that are important for the workflow.
Alapvető kérdések
A tervezetek megvitatása során csapatunk reméli, hogy az alapvető kérdésekkel kapcsolatos észrevételeket kapunk:
- A szerkesztésnek a szócikknél kell történnie (több kontextus)? Vagy egy külön erre a szerkesztési típusra szolgáló élményben (nagyobb fókusz, de nagyobb ugrás a szerkesztő használatához)?
- Mi történik, ha valaki módosítani szeretné a hivatkozás elérési útvonalát vagy szövegét? Megakadályozzuk, vagy hagyjuk, hogy egy szabványos szerkesztőhöz lépjen? Ez talán lehetőség arra, hogy megtanítsuk őket a vizuális szerkesztőre?
- Tudjuk, hogy alapvető fontosságú számunkra, hogy támogassuk a hagyományos szerkesztőeszközöket felfedező kezdőket. De mikor tesszük ezt? A strukturált feladatmegoldás során, emlékeztetőkkel, hogy a szerkesztő átmehet a szerkesztőbe? Vagy időszakosan, a befejezés mérföldköveinél, például bizonyos számú strukturált feladat elvégzése után?
- A "bot" a helyes kifejezés? Milyen más lehetőségek vannak? "Algoritmus", "számítógép", "automata", "gép" stb.?" Mi segíthetne jobban érzékeltetni, hogy a gépi ajánlások hibásak, és hogy fontos az emberi szerkesztés?
Mobile user testing: September 2020
Background
During the week of September 7, 2020, we used usertesting.com to conduct 10 tests of the mobile interactive prototypes, 5 tests each of Concepts A and B, all in English. By comparing how users interact with the two different approaches at this early stage, we wanted to better understand whether one or the other is better at providing users with good understanding and ability to successfully complete structured tasks, and to set them up for other kinds of editing afterward. Specific questions we wanted to answer were:
- Do users understand how they are improving an article by adding wikilinks?
- Do users seem like they will want to cruise through a feed of link edits?
- Do users understand that they're being given algorithmic suggestions?
- Do users make better considerations on machine-suggested links when they have the full context of the article (like in Concept A)?
- Do users complete tasks more confidently and quickly in a focused UI (like in Concept B)?
- Do users feel like they can progress to other, non-structured tasks?
Key findings
- The users generally were able to exhibit good judgment for adding links. They understood that AI is fallible and that they have to think critically about the suggestions.
- While general understanding of what the task would be ("adding links") was low at first, they understood it well once they actually started doing the task. Understanding in Concept B was marginally lower.
- Concept B was not better at providing focus. The isolation of excerpts in many cases was mistaken for the whole article. There were also many misunderstandings in Concept B about whether the user would be seeing more suggestions for the same term, for the same article, or for different articles.
- Concept A better conveyed expectations on task length than Concept B. But the additional context of a whole article did not appear to be the primary factor of why.
- As participants proceed through several tasks, they become more focused on the specific link text and destination, and less on the article context. This seemed like it could lead to users making weak decisions, and this is a design challenge. This was true for both Concepts A and B.
- Almost every user intuitively knew they could exit from the suggestions and edit the article themselves by tapping the edit pencil.
- All users liked the option to view their edits once they finished, either to verify or admire them.
- “AI” was well understood as a concept and term. People knew the link suggestions came from AI, and generally preferred that term over other suggestions. This does not mean that the term will translate well to other languages.
- Copy and onboarding needs to be succinct and accessible in multiple points. Reading our instructions is important, but users tended not to read closely. This is a design challenge.
Outcome
- We want to build Concept A for mobile, but absorbing some of the best parts of Concept B's design. These are the reasons why:
- User tests did not show advantages to Concept B.
- Concept A gives more exposure to rest of editing experience.
- Concept A will be more easily adapted to an “entry point in reading experience”: in addition to users being able to find tasks in a feed on their homepage, perhaps we could let them check to see if suggestions are available on articles as they read them.
- Concept A was generally preferred by community members who commented on the designs, with the reason being that it seemed like it would help users understand how editing works in a broader sense.
- We still need to design and test for desktop.
Ideas
The team had these ideas from watching the user tests:
- Should we consider a “sandbox” version of the feature that lets users do a dry run through an article for which we know the “right” and “wrong” answers, and can then teach them along the way?
- Where and when should we put the clear door toward other kinds of editing? Should we have an explicit moment at the end of the flow that actively invites them copyedit or do another level task?
- It’s hard to explain the rules of adding a link before they try the task, because they don't have context. How might we show them the task a little bit, before they read the rules?
- Perhaps we could onboard the users in stages? First they learn a few of the rules, then they do some links, then we teach them a few more pointers, then they do more links?
- Should users have a cooling-off period after doing lots of suggestions really fast, where we wait for patrollers to catch up, so we can see if the user has been reverted?
Desktop mockups: October 2020
After designing, testing, and deciding on Concept A for mobile users, we moved on to thinking about desktop users. We again have the same question around Concepts A and B. The links below open interactive prototypes of each, which we are using for user testing.
- Concept A: the structured task takes place at the article, in the editor, using some of the existing visual editor components. This gives users greater exposure to the editing context and may make it more likely that they explore other kinds of editing tasks.
- Concept B: the structured task takes place on the newcomer homepage, essentially embedding the compact mobile experience into the page. Because the user doesn't have to leave the page, this may encourage them to complete more edits. They could also see their impact statistics increase as they edit.
We are user testing these designs during the week of October 23. See below for mockups showing the main interaction in each concept.
-
Mockup of Concept A
-
Mockup of Concept B
Outcome
The results of the desktop user tests led us to decide on Concept A for desktop for many of the same reasons we chose Concept A for mobile. The convenience and speed of Concept B did not outweigh the opportunity for Concept A to expose newcomers to more of the editing experience.
Terminology
"Add a link" is a feature in which human users interact with an algorithm. As such, it is important that user have a strong understanding that suggestions come from an algorithm and that they should be regarded with skepticism. In other words, we want the users to understand that their role is to evaluate the algorithm's suggestion and not to trust it to much. Terminology (i.e. the words we use to describe the algorithm) play an important role in building that understanding.
At first, we planned to use the terms "artificial intelligence" and "AI" to refer to the algorithm, but we eventually decided to use the term "machine". This may be a practice that gets adopted more broadly as multiple teams build more structured tasks that are backed by algorithms. Below is how we thought about this decision.
Background
As we build experiences that incorporate augmentation, we are thinking about the terminology to use when referring to suggestions that come from automated systems. If possible, we want to make a smart choice at the outset, to minimize changes and confusion later. For instance, we are looking at sentences in the feature like these:
- "Suggested links are machine-generated, and can be incorrect."
- "Links are recommended by machine, and you will decide whether to add them to the article."
Objectives
We want the terms we use to satisfy these objectives.
- Transparency: users should understand where recommendations come from, and we should be being honest with them.
- Human-in-the-loop: users should understand that their contributions improve recommendations in the future.
- Usability: copy should help users complete the tasks, not confuse or burden them with too much information.
- Consistency: we should use the same copy as much as possible to lower cognitive load.
Terms we considered
Term | Strengths | Weaknesses |
AI |
|
|
Machine |
|
|
Computer |
|
|
Algorithm |
|
|
Automated |
|
|
Methods and findings
- User testing: the Growth team tested "add a link" in English using the terms "artificial intelligence" and "AI". We found that users understood the term well and that English-speaking users understood that they should regard the output of AI with skepticism.
- Experts: we spoke to WMF experts in the fields of artificial intelligence and machine learning. They explained that the link recommendation is not truly "AI", in the way that the term is used in the industry today. They explained that by using that term, we may be over-inflating our work and giving users a false sense of the intelligence of the algorithm. Experts preferred the term "machine", as it would accurately describe the link recommendation algorithm as well as be broad enough to describe almost any other kind of algorithm we might use for structured tasks.
- Multi-lingual community members: we spoke to about seven multilingual colleagues and ambassadors about the terms that would make the most sense in their languages. Not all languages have a short acronym for "AI"; many have long translations. The consensus was that "machine" made good sense in most languages and would be easy to translate.
Result
We are going to use the term "machine" to refer to the link recommendation algorithm, e.g. "Suggested links are machine-generated". See screenshots below to see one of the places where the terminology changed based on this decision.
-
Onboarding screen using the term "AI"
-
Onboarding screen using the term "machine"
Measurement
Hypotheses
The “add a link” workflow structures the process of adding wikilinks to a Wikipedia article, and assists the user with artificial intelligence to point out the clearest opportunities for adding links. Our hypothesis with the “add a link” workflow is that such a structured editing experience will lower the barrier to entry and thereby engage more newcomers, and more kinds of newcomers than an unstructured experience. We further hypothesize that newcomers with the workflow will complete more edits in their first session, and be more likely to return to complete more.
Below are the specific hypotheses we seek to validate. These govern the specifics around which data we'll collect and how we'll analyze it.
- The "add a link" structured task increases our core metrics of activation, retention, and productivity.
- “Add a link” edits are more likely to be successful than unstructured suggested edits, meaning that a user completes the task and saves the edit. They are also more likely to be constructive, meaning that the edit was not reverted, than unstructured suggested edits.
- Users seem to understand this task more than unstructured tasks.
- Users who start with "add a link" will move on to other kinds of tasks, instead of staying siloed (the latter being a primary community concern).
- The perceived quality of the link recommendation algorithm will be high, both from the users who make "add a link" edits and the communities who review those edits.
- Users who get “add a link” and who primarily use/edit wikis on mobile see a larger increase in the effects on retention and productivity relative to desktop users.
Experiment Plan
A randomly selected half of users who get the Growth features will get "add a link" tasks, and the other randomly selected half will get unstructured link tasks. We prefer to give users maximum exposure to these tasks and will therefore not give these users any copyedit tasks by default. In other words, for the purposes of this experiment, we’ll change the default difficulty filters from “links” and “copyedit” to just “links”. For wikis that don’t have unstructured link tasks, all those users get “add a link” and in that case we’ll exclude that wiki from the experiment.
We plan to continue to have a Control group that does not get access to the Growth features, which is a randomly selected 20% of new registrations.
- Group A: users get “add a link” as their only default task type.
- Group B: users get unstructured link task as their only default task type.
- Group C: control (no Growth features)
The experiment will run for a limited time, most likely between four to eight weeks. In practice the experiment will start with our four pilot wikis. After two weeks, we will analyze the leading indicators below to decide whether to extend the experiment to the rest of the Growth wikis.
Experiment Analysis and Findings
After completion of "Add a link" Experiment Analysis, we can conclude that the "add a link" structured task improves outcomes for newcomers over both a control group that did not have access to the Growth features as well as the group that had the unstructured "add links" tasks, particularly when it comes to constructive (non-reverted) edits. The most important points are:
- Newcomers who get the Add a Link structured task are more likely to be activated (i.e. make a constructive first article edit).
- They are also more likely to be retained (i.e. come back and make another constructive article edit on a different day).
- The feature also increases edit volume (i.e. the number of constructive edits made across the first couple weeks), while at the same time improving edit quality (i.e. the likelihood that the newcomer's edits are reverted).
Leading Indicators and Plan of Action
We are at this point fairly certain that Growth features are not detrimental to the wiki communities. That being said, we also want to be careful when experimenting with new features. Therefore, we define a set of leading indicators that we will keep track of during the early stages of the experiment. Each leading indicator comes with a plan of action in case the defined threshold is reached, so that the team knows what to do.
Indicator | Plan of Action |
---|---|
Revert rate | This suggests that the community finds the Add a Link edits to be unconstructive. If the revert rate for Add a Link is significantly higher than that of unstructured link tasks, we will analyze the reverts in order to understand what causes this increase, then adjust the task in order to reduce the likelihood of edits being reverted. |
User rejection rate | This can indicate that we are suggesting a lot of links that are not good matches. If the rejection rate is above 30%, we will QA the link recommendation algorithm and adjust thresholds or make changes to improve the quality of the recommendations. |
Task completion rate | This might indicate that there’s an issue with the editing workflow. If the proportion of users who start the Add a Link task and complete it is lower than 75%, we investigate where in the workflow users leave and deploy design changes to enable them to continue. |
Analysis and Findings
We collected data on usage of Add a Link from deployment on May 27, 2021 until June 14, 2021. This dataset excluded known test accounts, and does not contain data from users who block event logging (e.g. through their ad blocker).
This analysis categorizes users into one of two categories based on when they registered. Those who registered prior to feature deployment on May 27, 2021 are labelled "pre-deployment", and those who registered after deployment are labelled "post-deployment". We do this because users in the "post-deployment" group are randomly assigned (with 50% probability) into either getting Add a Link or the unstructured link task. Users in the "pre-deployment" group have the unstructured link task replaced by Add a Link. By splitting into these two categories, we're able to make meaningful comparisons between Add a Link and the unstructured link task, for example when it comes to revert rate.
Revert rate: We use edit tags to identify edits and reverts, and reverts have to be done within 48 hours of the edit. The latter is in line with common practices for reverts.
User registration | Task type | N edits | N reverts | Revert rate |
---|---|---|---|---|
Post-deployment | Add a Link | 290 | 28 | 9,7% |
Unstructured | 63 | 22 | 34,9% | |
Pre-deployment | Add a Link | 958 | 49 | 5,1% |
For the post-deployment group, a Chi-squared test of proportions finds the difference in revert rate to be statistically significant (). This means that the threshold described in the leading indicator table is not met.
Rejection rate: We define an "edit session" as reaching the edit summary or skip all dialogue, at which point we count the number of links that were accepted, rejected, or skipped. Users can reach this dialogue multiple times, because we think that choosing to go back and review links again is a reasonable choice.
User registration | N accepted | % | N rejected | % | N skipped | % | N total |
---|---|---|---|---|---|---|---|
Post-deployment | 597 | 72,4 | 125 | 15,2 | 103 | 12,5 | 825 |
Pre-deployment | 1 464 | 65,1 | 595 | 26,5 | 189 | 8,4 | 2 248 |
2 061 | 67,1 | 720 | 23,4 | 292 | 9,5 | 3 073 |
The threshold in the leading indicator table was a rejection rate of 30%, and this threshold has not been met.
Over-acceptance rate: This was not part of the original leading indicators, but we ended up checking for it as well in order to understand whether users were clicking "accept" on all the links and saving those edits. We reuse the concept of an "edit session" from the rejection rate analysis, and count the number of users who only have sessions where they accepted all links. In order to understand whether these users make many edits, we measure this for all users as well as for those with five or more edit sessions. In the table below, the "N total" column shows the total number of users with that number of edit sessions, and "N accepted all" the number of users who only have edit sessions where they accepted all suggested links.
User registration | N total | N accepted all | % | |
---|---|---|---|---|
Post-deployment | ≥1 edit | 96 | 31 | 32,3 |
≥5 edits | 6 | 0 | 0,0 | |
Pre-deployment | ≥1 edit | 64 | 10 | 15,6 |
≥5 edits | 19 | 1 | 5,3 |
We find that some users only have sessions where they accepted all links, but these users do not typically continue to make Add a Link edits. Instead, users who make additional edits start rejecting or skipping links as needed.
Task completion rate: We define "starting a task" as having an impression of "machine suggestions mode". In other words, the user is loading the editor with an Add a Link task. "Completing a task" is defined as clicking to save an edit, or confirming that all suggested links were skipped.
User registration | N Started a Task | N Completed 1+ Tasks | % |
---|---|---|---|
Post-deployment | 178 | 96 | 53,9 |
Pre-deployment | 101 | 64 | 63,4 |
279 | 160 | 57,3 |
The threshold defined in the leading indicator table is "lower than 75%", and this threshold has been met. In this case, we're planning to do follow-up analysis to understand more about the tasks, e.g. if they had a low number of suggested links, or if this happens on specific wikis or platforms.
Rejection Reasons
We've analyzed data on why users reject suggested links, focusing on newcomers to help us understand how they learn what constitutes good links in Wikipedia. In this analysis, we used rejections from January and February 2022, and restricted it to actions made within 7 or 28 days since registration. There was no significant difference in patterns between the two, and the data reported here uses the 28 day window. The data was split by wiki, platform (desktop or mobile) and bucketed by the number of Add a Link edits the user had made. We used a logarithmic bucketing scheme with 2 as the base, because that gives us a fair number of buckets early in a user's life while at the same time being easy to understand since the limits double each time.
Platform | Rejection reason | N | % |
---|---|---|---|
Desktop | Almost everyone knows what it is | 2 732 | 53,0% |
Linking to wrong article | 1 377 | 26,7% | |
Other | 688 | 13,0% | |
Text should include more or fewer words | 378 | 7,3% | |
Mobile | Almost everyone knows what it is | 1 835 | 53,3% |
Linking to wrong article | 791 | 23,0% | |
Other | 484 | 14,1% | |
Text should include more or fewer words | 271 | 7,9% | |
Undefined | 62 | 1,8% |
The distribution of these reasons is generally the same across all wikis, platforms, and number of Add a Link edits made. For some combinations of these features, we run into the issue of having few data points available (e.g. because some wikis lean strongly to usage of one platform) and that might result in a somewhat different distribution (e.g. just ones marked "Text should include more or fewer words"). In general, we have a lot of data for users with few edits as that's what most users are.
One thing we do appear to see is that for some wikis the usage of "Other" decreases as the number of Add a Link edits made increases. We interpret this to mean that "Other" might be a catchall/safe category for less experienced users, and that as they become more experienced and confident in labelling a link they'll use a different category.
Engineering
Link recommendation algorithm
See this page for an explanation of the link recommendation algorithm and for statistics around its accuracy. In short, we believe that users will experience an accuracy around 75%, meaning that 75% of the suggestions they get should be added. It is possible to tune this number, but the higher the accuracy is, the fewer candidate link we will be able to recommend. After the feature is deployed, we can look at revert rates to get a sense of how to tune that parameter.
For a detailed understanding of how the algorithm functions and is evaluated, see this research paper.
Link recommendation service backend
To follow along with engineering progress on the backend "add link" service, please see this page on Wikitech.
Articles selection
Articles are selected based on the topic(s) the use chooses. Then articles with a low ratio of links compared to the number of words are selected. A small amount of randomness was added to it so that not every article suggested look the same. The formula is documented on Phabricator.
Deployment
On May 27, 2021, we deployed the first iteration of this task to our four pilot wikis: Arabic, Czech, Vietnamese, and Bengali Wikipedias. It is available to half of new accounts, as described above. All accounts created before the deployment will also have the feature available. After two weeks, we will analyze our leading indicators to determine if any quick changes need to be made. After about four weeks, we will use data and community feedback to determine whether and how to deploy the feature to more wikis.
Since the initial deployment to our pilot wikis, the Growth team has gathered extensive community feedback and data about the usage and value of Add a Link. We then used those learnings to make improvements to the feature so that newcomers would have a better experience and experienced editors would see higher quality edits. We have completed the improvements, and communities are now using iteration 2 of Add a Link.
The deployment to all Wikipedias was completed at the end of 2023, with only few wikis missing: German and English as they require specific community engagement, and very small Wikipedias where there is no critical mass to have enough links.